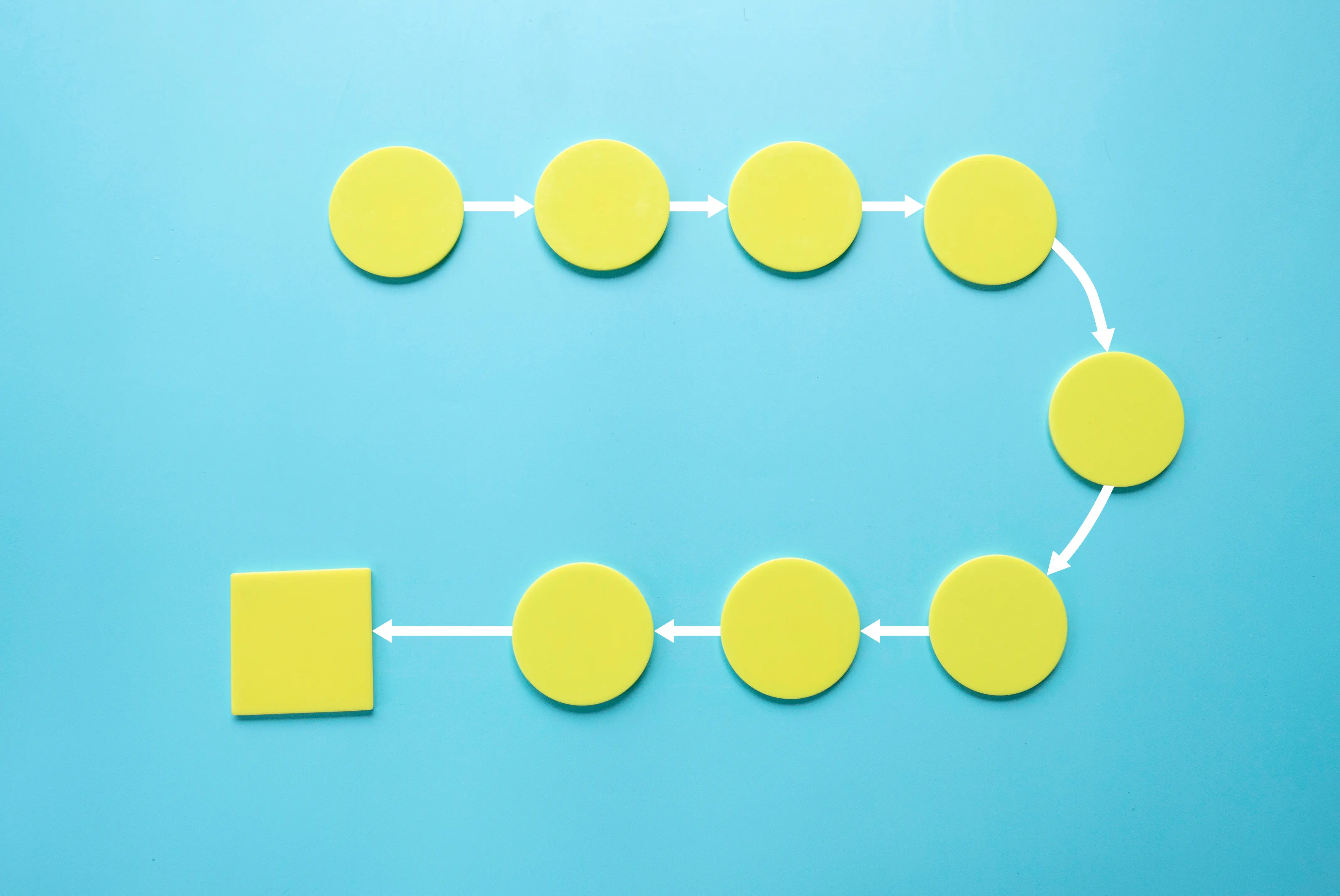
I. Introduction
The integration of Artificial Intelligence (AI) into cloud computing environments represents a significant inflection point in business process automation. As data analysts and workflow optimization specialists, we observe that cloud platforms provide the necessary scalability and flexibility, while AI introduces intelligence and adaptability previously unattainable. This synergy is fundamentally reshaping how organizations approach operational efficiency. The data clearly indicates a growing reliance on these combined technologies; industry analysis suggests that the market for AI in cloud computing is expanding rapidly, reflecting its increasing importance.
The significance of AI-powered workflows extends beyond simple task automation. We are now witnessing the emergence of intelligent systems capable of complex decision-making, predictive analysis, and continuous self-improvement within cloud infrastructures. These capabilities allow businesses to not only streamline existing processes but also to unlock entirely new operational models and competitive advantages. Measuring the impact requires rigorous analysis, focusing on metrics like processing speed, error reduction, and resource utilization improvements.
This article will delve into specific, innovative use cases where AI automation is making a measurable difference in cloud-based workflows. We will examine practical applications across various business functions, from document processing and customer service to finance and HR. By analyzing these examples, readers will gain a data-driven understanding of how to leverage cloud AI solutions for tangible improvements in efficiency, accuracy, and strategic decision-making within their own operations.
II. Understanding AI Automation in Cloud Workflows
AI automation in the context of cloud workflows refers to the use of artificial intelligence techniques—such as machine learning (ML), natural language processing (NLP), and computer vision—to automate tasks and decision-making processes hosted on cloud infrastructure. Key components typically include cloud platforms (like AWS, Azure, Google Cloud), AI services offered by these platforms or third-party providers, automation tools (like Zapier, Make.com, n8n), and the specific algorithms tailored to the workflow. The core idea is to move beyond rule-based automation to systems that can learn, adapt, and handle variability.
Combining AI with cloud-based processes yields substantial benefits, quantifiable through careful measurement. The inherent scalability of the cloud allows AI models to process vast datasets and handle fluctuating workloads without significant upfront infrastructure investment, leading to optimized resource allocation. Furthermore, cloud platforms facilitate seamless integration of various AI tools and services, creating cohesive, end-to-end automated workflows. Analysis consistently shows that this combination results in reduced operational costs and accelerated processing times compared to traditional, on-premise automation efforts.
The current state of AI automation technology is characterized by increasing accessibility and sophistication. Pre-trained models and low-code/no-code automation platforms are lowering the barrier to entry, enabling organizations without deep AI expertise to implement intelligent workflows. Advancements in ML algorithms, particularly deep learning, are enhancing capabilities in areas like pattern recognition and prediction. Ongoing A/B testing and performance monitoring reveal continuous improvements in model accuracy and efficiency, driving wider adoption across industries seeking digital transformation through intelligent automation.
III. Revolutionary Use Case #1: Intelligent Document Processing
One of the most impactful applications of AI automation in cloud workflows is Intelligent Document Processing (IDP). This involves using AI, primarily NLP and computer vision, to automatically classify, route, extract, and validate information from various document types, such as invoices, contracts, and forms. Cloud-based IDP systems can handle diverse formats and layouts, learning over time to improve accuracy, a significant advantage over traditional OCR methods which often struggle with variability. Performance metrics show IDP can reduce manual data entry efforts by up to 80%.
Automated document classification and routing form the initial stage of many IDP workflows. AI models analyze document content and structure to determine the document type and its relevant business context. Based on this classification, the system automatically routes the document to the appropriate department or next step in the workflow, significantly speeding up internal processes. Testing reveals that AI-driven routing achieves higher accuracy rates (often exceeding 95%) compared to manual sorting or simple rule-based systems, especially in high-volume environments.
A compelling real-world example is found in insurance claims processing. Insurers receive vast quantities of documents – claim forms, medical reports, repair estimates, photographs. An AI-powered cloud workflow can ingest these documents, classify them, extract key data points (like policy number, claimant details, incident description, damage assessment), and validate this information against existing records. This automation drastically reduces claim handling time; case studies indicate processing times cut by 30-50%, leading to faster settlements and improved customer satisfaction. Implementing such a system often involves integrating cloud storage, AI services (like AWS Textract or Google Document AI), and workflow automation platforms like Zapier to connect the different stages seamlessly.
IV. Revolutionary Use Case #2: Predictive Customer Service
AI automation is revolutionizing customer service operations hosted in the cloud, moving beyond simple chatbots to predictive and proactive support systems. By analyzing historical support data, communication patterns, and customer profiles, AI can anticipate customer needs and potential issues. This predictive capability allows businesses to address problems before they escalate, significantly enhancing the customer experience. Data analysis from early adopters shows a correlation between predictive service initiatives and increased customer retention rates.
A key application is AI-powered ticket routing and prioritization. When a customer query arrives (via email, chat, or portal), AI algorithms analyze the content, sentiment, and customer history to determine the urgency and complexity of the issue. The system then automatically routes the ticket to the best-suited agent or department, ensuring faster and more effective resolution. Measurement of these systems demonstrates a reduction in average handling time by 15-25% and ensures critical issues receive immediate attention, improving overall service levels.
Furthermore, AI contributes through automated response generation and sentiment analysis integration. For common queries, AI can draft contextually relevant responses, which agents can review and send, saving considerable time. Sentiment analysis, applied in real-time to customer interactions, provides agents with insights into the customer's emotional state, enabling more empathetic and effective communication. An e-commerce case study implementing cloud-based AI for customer support observed a 20% improvement in first-contact resolution and a 15-point increase in Customer Satisfaction (CSAT) scores by combining intelligent routing, automated response suggestions, and sentiment analysis within their cloud CRM and support platform.
V. Revolutionary Use Case #3: Smart Content Operations
AI automation is increasingly integral to optimizing content operations within cloud-based environments, encompassing creation, distribution, and quality assurance. Leveraging AI tools hosted in the cloud allows marketing and content teams to scale their efforts and improve content performance significantly. Automated content creation tools, powered by generative AI models like GPT, can assist in drafting articles, social media posts, and product descriptions based on specified inputs and data feeds. While human oversight remains crucial, analysis shows these tools can accelerate initial draft creation by over 50%.
AI also plays a critical role in optimizing content for discovery and engagement through data-driven insights. AI algorithms analyze performance data, audience behavior, and competitor content to recommend optimal topics, keywords, formats, and publishing times. This allows for more strategic content planning and refinement. Furthermore, AI-driven content distribution involves automatically tailoring and pushing content across various channels (social media, email, ad platforms) based on audience segmentation and predicted engagement, maximizing reach and impact. A/B testing different AI-driven distribution strategies often reveals significant uplifts in click-through rates (CTRs) and conversion rates.
Quality assurance in content operations can also be enhanced through AI automation. AI tools can automatically check for grammatical errors, plagiarism, style guide adherence, and even brand voice consistency across large volumes of content. This frees up human editors to focus on higher-level aspects like narrative flow and strategic messaging. An example workflow using a platform like Make.com could involve triggering an AI writing assistant when a new content brief is added to a project management tool, sending the draft to an AI proofreading service, and then routing the reviewed content for final human approval, demonstrating a streamlined, cloud-native process that ensures both speed and quality, potentially reducing editorial review time by 30%.
VI. Revolutionary Use Case #4: Intelligent Financial Operations
Financial operations are prime candidates for AI automation within cloud workflows, offering substantial improvements in efficiency, accuracy, and risk management. Manual financial processes are often repetitive, time-consuming, and prone to error, making them ideal for intelligent automation solutions. Cloud-based AI enables scalable processing of financial data, sophisticated analysis, and integration with existing financial systems. The measurable impact includes significant reductions in processing costs and error rates.
Automated invoice processing is a prominent example. AI systems utilizing OCR and NLP can extract data from invoices received in various formats (PDF, scanned images, emails), validate the information against purchase orders and vendor records, and route invoices for approval and payment within a cloud accounting system. This automation drastically reduces manual data entry and accelerates the accounts payable cycle. Data from implementations shows invoice processing times can be reduced from days to hours, improving cash flow management and vendor relationships.
Beyond processing, AI excels in fraud detection and predictive financial forecasting. Machine learning models trained on historical transaction data can identify anomalous patterns indicative of fraudulent activity in real-time, flagging suspicious transactions far more effectively than rule-based systems. Analysis indicates AI-powered fraud detection systems can improve detection rates by 25% or more while reducing false positives. Similarly, AI algorithms can analyze vast datasets (market trends, historical performance, economic indicators) to generate more accurate financial forecasts, aiding strategic planning and budgeting. An implementation example could involve using n8n to connect a cloud accounting platform with an AI service that analyzes transaction data for anomalies and generates predictive cash flow reports, providing finance teams with timely, actionable insights.
VII. Revolutionary Use Case #5: HR Process Automation
Human Resources (HR) departments can leverage AI automation in cloud-based workflows to streamline numerous processes, improve candidate and employee experiences, and make more data-driven talent decisions. Cloud HR platforms integrated with AI capabilities offer powerful tools for managing the entire employee lifecycle more efficiently. The potential for reducing administrative overhead and improving the quality of hires is substantial, as indicated by early adoption metrics.
Resume screening and candidate matching represent a significant time-saving application. AI algorithms can parse thousands of resumes stored in a cloud database, extracting relevant skills, experience, and qualifications, and then match candidates to open job requisitions based on predefined criteria and learned patterns of successful hires. This allows recruiters to focus their efforts on the most promising candidates, significantly accelerating the hiring process. Studies show AI screening can reduce time-to-fill positions by up to 30% and improve the relevance of shortlisted candidates.
Other key areas include employee onboarding automation and performance analytics. AI can personalize the onboarding experience by automatically assigning relevant training modules, scheduling introductory meetings, and providing timely information through chatbots integrated into cloud HR portals. For performance management, AI can analyze performance data, feedback, and goal progression to identify trends, predict potential attrition risks, and suggest areas for employee development. A real-world implementation might involve using a cloud HR suite where AI automatically screens applicants, triggers personalized onboarding workflows upon hiring, and provides managers with predictive analytics on team performance, leading to more efficient HR operations and improved employee engagement metrics.
VIII. Best Practices for Implementation
Successfully implementing AI automation in cloud workflows requires careful planning and adherence to best practices, grounded in rigorous analysis. Selecting the right AI tools is paramount; this involves evaluating not only the capabilities of the AI models but also their integration potential with existing cloud infrastructure and workflows. Conducting pilot projects and A/B tests with different tools can provide empirical data on performance and ROI before committing to a large-scale deployment. Factors like data requirements, model trainability, scalability, and vendor support must be systematically assessed.
Integration considerations are critical for creating seamless end-to-end automated processes. Utilizing cloud-native integration platforms (iPaaS) or workflow automation tools like Zapier, Make.com, or n8n can simplify connecting disparate systems (e.g., CRM, ERP, AI services, cloud storage). Defining clear API contracts and data mapping protocols is essential for reliable data flow. Thorough integration testing, including load testing and error handling scenarios, is necessary to ensure the automated workflow is robust and performs as expected under various conditions, aiming for minimal disruption to existing operations.
Security and compliance measures cannot be overlooked, especially when handling sensitive data in the cloud. Implementing robust access controls, data encryption (both at rest and in transit), and regular security audits are fundamental. Ensuring compliance with relevant regulations (like GDPR or HIPAA) requires careful design of data handling processes within the automated workflow. Furthermore, developing effective scaling strategies from the outset is crucial. This involves leveraging the elasticity of cloud resources, designing modular workflows that can be easily expanded, and continuously monitoring performance metrics (like processing time, resource consumption, error rates) to identify bottlenecks and optimize resource allocation for sustained efficiency as workloads grow.
IX. Common Challenges and Solutions
Despite the significant benefits, implementing AI automation in cloud workflows presents challenges that require analytical approaches to overcome. Data quality issues are frequently encountered; AI models are only as good as the data they are trained on. Inconsistent, incomplete, or biased data can lead to inaccurate predictions and flawed automation. The solution involves implementing rigorous data governance practices, including data cleansing, validation, and augmentation techniques, coupled with ongoing monitoring of data pipelines to ensure data integrity is maintained at a high standard (e.g., >99% accuracy).
Integration complexities often arise when connecting legacy systems with modern cloud AI services. Differences in data formats, communication protocols, and API limitations can hinder seamless workflow automation. Addressing this requires careful planning, potentially using middleware or iPaaS solutions to bridge the gaps, and conducting thorough end-to-end testing. Documenting integration points and dependencies meticulously helps in troubleshooting and future modifications. Allocating sufficient resources for integration testing can reduce deployment failures by a significant margin.
Cost considerations are another key challenge. While cloud AI offers scalability, the costs associated with API calls, data storage, model training, and specialized expertise can accumulate. A detailed cost-benefit analysis, including projected ROI based on efficiency gains and error reduction, is essential before embarking on implementation. Optimizing workflows to minimize unnecessary AI processing and leveraging tiered pricing models can help manage expenses. Finally, performance optimization is an ongoing task. Monitoring key performance indicators (KPIs), regularly retraining models with new data, and conducting A/B tests on different algorithms or workflow configurations are crucial for ensuring the automation remains effective and efficient over time, aiming for continuous improvement in target metrics like speed and accuracy.
X. Future Trends and Opportunities
The field of AI automation in cloud workflows is rapidly evolving, with emerging capabilities poised to unlock even greater potential for businesses. We anticipate advancements in areas like explainable AI (XAI), which will provide clearer insights into how AI models arrive at decisions, fostering greater trust and facilitating easier debugging and compliance verification. The integration of more sophisticated reinforcement learning techniques could enable workflows that autonomously optimize themselves based on real-time feedback and changing conditions, leading to truly adaptive business processes.
Predicted developments also include the rise of hyperautomation – the orchestrated use of multiple AI, machine learning, robotic process automation (RPA), and other automation technologies to automate as many business processes as possible. Cloud platforms will serve as the central hub for these integrated automation ecosystems. Furthermore, advancements in edge computing integrated with cloud AI will enable faster processing and decision-making closer to the data source, particularly relevant for IoT and real-time operational workflows. Analysis suggests these trends will further accelerate digital transformation initiatives.
Preparing for these future innovations requires a proactive and analytical approach. Organizations should foster a culture of continuous learning and experimentation, encouraging teams to explore new AI tools and techniques within controlled environments. Investing in data infrastructure and governance is crucial to ensure readiness for more advanced AI applications. Building modular and flexible cloud architectures will facilitate the integration of future AI capabilities with minimal disruption. Staying informed about industry benchmarks and performance metrics will allow businesses to strategically adopt innovations that offer quantifiable competitive advantages.
XI. Conclusion
In summary, the integration of AI automation into cloud-based workflows presents transformative opportunities across diverse business functions. We've examined innovative use cases demonstrating significant, measurable improvements in efficiency, accuracy, and strategic capability – from intelligent document processing that slashes handling times to predictive customer service enhancing retention, smart content operations boosting engagement, intelligent financial operations improving accuracy and detecting fraud, and streamlined HR processes reducing time-to-hire. The data consistently points towards substantial ROI when these technologies are implemented thoughtfully.
The key takeaways emphasize the synergistic power of AI and the cloud, enabling scalable, intelligent automation that adapts to complex, dynamic business needs. Successful implementation hinges on careful tool selection, robust integration strategies, stringent security and compliance measures, and proactive management of challenges like data quality and cost. Analyzing performance metrics and embracing best practices are crucial for realizing the full potential of these cloud AI solutions.
For organizations looking to harness these capabilities, the next steps involve identifying high-impact processes suitable for automation, conducting pilot projects to validate potential benefits, and developing a strategic roadmap for broader implementation. Leveraging workflow automation platforms and cloud AI services effectively requires both technical understanding and a clear focus on measurable business outcomes. Additional resources, including case studies and technical documentation from leading cloud providers and automation platforms, can provide further guidance.
XII. Call to Action
Ready to optimize your own cloud-based workflows with AI automation?
- Download our Free Workflow Templates designed to kickstart your intelligent automation journey in areas like document processing and customer service analysis.
- Schedule a Free Consultation with our workflow optimization analysts to discuss your specific challenges and identify high-ROI automation opportunities based on data-driven assessments.
- Sign up for The AI Automation Guide Newsletter to receive regular updates on the latest AI automation trends, tools, performance benchmarks, and implementation strategies directly in your inbox.