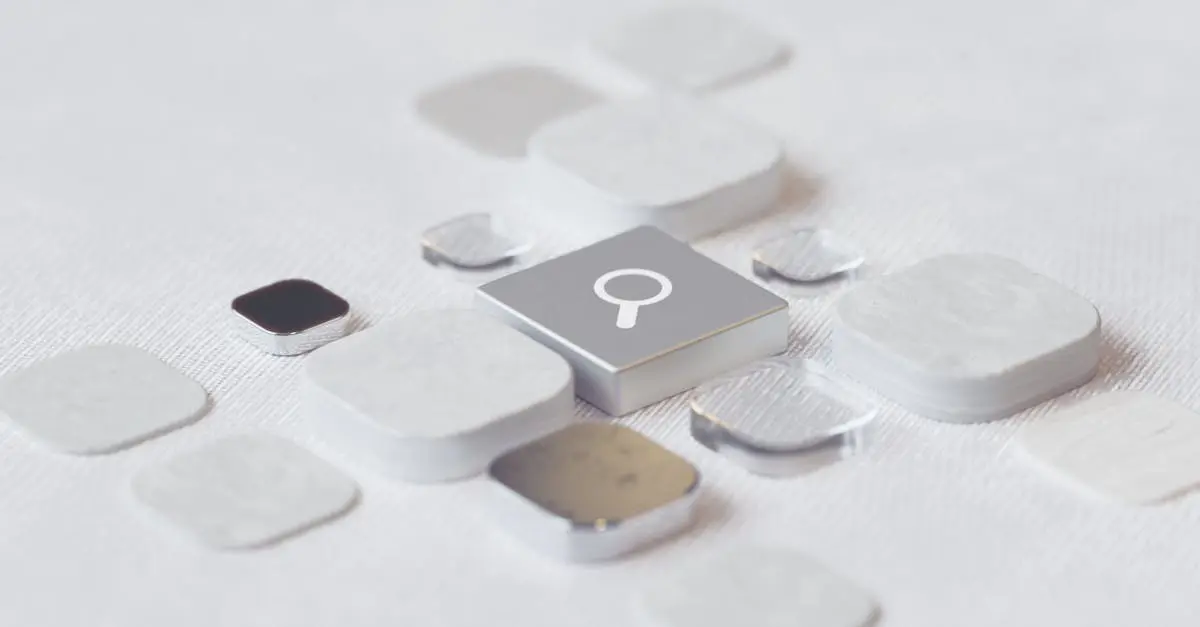
The daily deluge of emails presents a significant operational challenge for professionals across industries. Data suggests the average worker spends 28% of their workweek managing email, a substantial drain on productivity that hinders focus on core responsibilities. This constant influx often leads to missed opportunities, delayed responses, and increased stress levels, impacting overall business efficiency. As analysts focused on workflow optimization, we see this not just as an annoyance, but as a critical bottleneck demanding a data-driven solution.
Fortunately, the integration of Artificial Intelligence (AI) into automation platforms offers a powerful method for transforming inbox management. By leveraging AI, we can move beyond simple rule-based filtering to create truly intelligent email workflows. These systems can understand context, prioritize tasks, and even assist with communication, leading to measurable improvements in efficiency and organization.
This post will explore how AI automation can revolutionize your email handling processes. We will delve into the underlying technologies, examine essential tools, provide practical steps for implementation, and discuss methods for measuring the success of these automated systems. The objective is to provide a clear, analytical framework for reclaiming valuable time and reducing the cognitive load associated with managing a high volume of emails.
Understanding AI-Powered Email Automation
What elevates standard email automation to "intelligent" automation is the incorporation of AI capabilities like Natural Language Processing (NLP) and Machine Learning (ML). These technologies allow automation tools to interpret the content and context of emails, rather than just relying on predefined keywords or sender addresses. This understanding enables more nuanced and effective workflow execution, adapting over time as it processes more data.
Key capabilities unlocked by AI in email management include semantic analysis for accurate categorization, sentiment analysis to gauge urgency or tone, and predictive modeling for anticipating user needs. For instance, an AI system can differentiate between a routine newsletter and an urgent client request, even if both contain similar keywords. Studies like those highlighted by McKinsey on the economic potential of generative AI point towards significant productivity gains, estimating that generative AI could automate tasks occupying 60 to 70 percent of employees' time, with email management being a prime candidate.
Common use cases demonstrate the practical value of this technology. AI can automatically sort incoming emails into highly specific folders, flag messages requiring immediate attention based on content analysis, draft context-aware replies, and extract key information to populate other business systems like CRMs or task managers. This moves beyond simple filtering towards proactive inbox assistance, directly impacting workflow efficiency and data accuracy.
Essential AI Tools for Email Automation
Several categories of tools are fundamental for building effective AI-driven email workflows. Email classification and sorting tools utilize machine learning algorithms to automatically categorize incoming messages based on content, sender, and other contextual clues. These tools learn user preferences and organizational systems over time, becoming increasingly accurate in routing emails to appropriate labels or folders, significantly reducing manual sorting effort.
Priority inbox systems represent another critical component, employing AI to analyze emails and assign urgency scores. These systems often consider factors like sender importance, keywords indicating deadlines or critical issues, and historical interaction patterns. The result is an inbox that surfaces the most crucial communications first, ensuring that high-priority items receive prompt attention while less critical messages are deferred.
Furthermore, smart reply and composition assistants, often powered by generative AI models, are becoming increasingly prevalent. Tools like the AI email assistants discussed by Zapier can suggest relevant responses, summarize long email threads, or even draft entire emails based on brief prompts. Integration platforms such as Zapier and Make.com are essential for connecting these specialized AI tools with your email client and other productivity software, enabling seamless, automated workflows across your entire digital workspace.
Setting Up Basic AI Email Workflows
Implementing foundational AI email workflows begins with effective classification and priority management. These initial steps provide immediate value by reducing inbox clutter and highlighting critical communications.
A. Email Classification
The first step involves setting up automated folders or labels within your email client that correspond to key projects, clients, or categories of communication. Once this structure is in place, AI tools can be configured to automatically sort incoming emails. This typically involves connecting your email account to an AI classification tool or an integration platform with AI capabilities.
Training the AI is a crucial phase where the system learns to recognize patterns associated with different email types. Initially, this might involve manually correcting misclassified emails or providing examples for each category. Over time, the AI model refines its understanding, achieving high accuracy in automatic sorting based on nuanced content analysis, not just simple keyword matching.
Creating specific rules within the automation tool enhances the AI's effectiveness. For example, a rule could dictate that all emails containing invoice-related terms from known vendor domains are automatically moved to an "Accounts Payable" folder and flagged for review. These rules, combined with the AI's learning capabilities, form the backbone of an efficient automated classification system, demonstrably reducing the time spent on manual inbox organization.
B. Priority Management
Implementing AI-based priority scoring requires configuring a system to analyze incoming emails and assign a level of importance. This often involves setting parameters within an AI tool or platform, defining what constitutes a high-priority message for your specific context. Factors might include specific sender domains, keywords related to urgent matters, or even sentiment analysis indicating frustration or immediate need.
Setting up VIP sender recognition is a common and effective technique. You can designate key contacts (clients, managers, critical partners) whose emails should always be flagged as high priority. The AI system ensures these messages are prominently displayed, perhaps through a dedicated "Priority Inbox" view or specific visual cues, minimizing the risk of overlooking critical communications.
Automated filtering of promotional content and newsletters is another key aspect of priority management. AI tools excel at identifying bulk mailings and non-essential communications, automatically moving them to designated folders or applying labels that deprioritize them in the main inbox view. This filtering significantly declutters the primary inbox, allowing users to focus their attention on emails requiring action or response, thereby improving focus and reducing cognitive load associated with sifting through irrelevant messages.
Advanced Automation Techniques
Once basic classification and prioritization are established, more sophisticated automation techniques can yield further efficiency gains. These often involve connecting multiple steps and integrating email workflows with other business processes.
A. Multi-step Workflows
Advanced automation moves beyond single trigger-action rules to encompass multi-step workflows involving conditional logic. For instance, an incoming email identified by AI as a customer support query could trigger a sequence: first, log the query in a helpdesk system; second, assign it to a specific support agent based on keywords; and third, send an automated acknowledgement to the customer. Platforms like Make.com offer robust capabilities for building such email automation sequences.
Creating conditional logic ("if-then-else" statements) within these workflows allows for dynamic handling of different scenarios. An example workflow might be: IF an email contains an attachment AND the sender is in the CRM database, THEN save the attachment to a specific cloud storage folder AND update the contact record in the CRM. ELSE, IF the email is from an unknown sender, THEN flag it for manual review. This level of sophistication enables automation to handle a wider range of tasks accurately.
These complex workflows are particularly valuable for handling intricate email scenarios common in sales, project management, or customer service. By chaining together multiple actions and applying conditional rules based on AI analysis, businesses can automate processes that previously required significant manual intervention, freeing up employee time for higher-value activities. The potential impact aligns with observations from sources like Harvard Business Review on how generative AI is changing work, suggesting a fundamental shift in task distribution.
B. Integration with Other Productivity Tools
Integrating AI email workflows with calendar applications enables seamless scheduling and time management. For example, an AI could identify an email requesting a meeting, extract proposed times, check calendar availability, and even draft a reply suggesting suitable slots or confirming the appointment. This eliminates the back-and-forth often required for scheduling.
Connections to task management systems allow AI to automatically create tasks based on email content. An email discussing action items from a meeting could trigger the creation of corresponding tasks in a system like Asana or Trello, assigned to the relevant individuals with deadlines extracted from the email body. This ensures accountability and prevents action items from being lost in the inbox.
Synchronizing email interactions with Customer Relationship Management (CRM) systems provides a holistic view of customer communications. An AI workflow can automatically log relevant emails under the corresponding contact record in the CRM, attach important documents, or update contact details based on email signatures. This ensures that sales and support teams have access to the latest interaction history, improving customer service and relationship management.
Step-by-Step Tutorial: Building Your First AI Email Workflow
Creating your initial AI-powered email workflow involves a structured approach, starting with platform selection and progressing through testing and optimization. Let's outline the key steps from an analytical perspective.
First, choose the right automation platform based on your technical requirements and existing toolset. Consider platforms like Zapier or Make.com, evaluating their AI capabilities, available integrations (email client, CRM, etc.), pricing models, and ease of use. Analyze which platform offers the specific AI functions needed for your target workflow, such as text classification, entity extraction, or sentiment analysis.
Next, set up the necessary connections by authorizing the chosen platform to access your email account and any other relevant applications (e.g., Google Workspace, Microsoft 365, Slack, CRM). This typically involves OAuth authentication or API key generation. Ensure that permissions granted are appropriate and adhere to your organization's security policies, verifying data handling practices.
With connections established, create the workflow by defining a trigger (e.g., "New Email Received") and subsequent actions, incorporating AI modules where needed. For a simple classification workflow: Trigger = New Email -> Action 1 = Analyze email content with AI -> Action 2 = Apply label/move to folder based on AI category. Test the workflow rigorously with various email types (different senders, content, attachments) to validate its accuracy and logic. A/B testing different AI prompts or classification models can help identify the most effective configuration.
Finally, implement robust monitoring and plan for ongoing optimization. Track key performance indicators (KPIs), such as the percentage of emails correctly classified or the reduction in manual sorting time (more on this in the Measuring Success section). Regularly review workflow logs to identify errors or areas for improvement, adjusting rules and AI parameters based on performance data to maintain high efficiency.
Best Practices and Tips
To maximize the benefits of AI email automation, adhering to best practices is crucial for sustained efficiency and reliability. Continuous monitoring and refinement are key analytical principles in workflow optimization.
Maintaining workflow efficiency requires periodic review and adjustment. Set baseline metrics before implementation and track performance over time. If accuracy degrades (e.g., increased misclassification), retrain the AI model or adjust workflow rules. Regularly prune unused or redundant workflows to prevent system bloat and maintain clarity.
Avoiding common pitfalls involves careful planning and realistic expectations. Don't attempt to automate overly complex or ambiguous tasks initially; start with clear, high-volume processes. Ensure fallback mechanisms are in place for emails the AI cannot confidently process, routing them for manual review rather than risking incorrect automated actions. Over-reliance on automation without human oversight, especially for sensitive communications, can lead to errors.
Security considerations are paramount when granting third-party tools access to email data. Choose reputable platforms with strong security protocols and data encryption. Understand data residency and compliance requirements (e.g., GDPR, CCPA) relevant to your operations. Regularly review granted permissions and utilize features like multi-factor authentication to secure platform access.
Regular maintenance and updates are essential for long-term success. Keep platform integrations and AI models updated to benefit from the latest features and security patches. Periodically audit workflows to ensure they align with current business processes and objectives, making data-driven adjustments as needed.
Measuring Success
Quantifying the impact of AI email automation is essential for demonstrating value and guiding optimization efforts. A data-driven approach relies on tracking relevant metrics and analyzing workflow performance.
Key metrics to track include time saved (estimated reduction in manual email processing time), workflow accuracy (e.g., percentage of emails correctly classified or prioritized), response time improvement (for workflows involving automated replies or task creation), and inbox volume reduction (number of emails automatically filed or archived). Establishing baseline measurements before implementation allows for clear quantification of improvements. For instance, tracking the average time spent daily on email before and after automation provides a direct measure of efficiency gains.
Analyzing workflow effectiveness involves examining operational data generated by the automation platform. Review logs to identify successful executions, errors, and instances requiring manual intervention. This analysis can reveal bottlenecks, common failure points, or specific types of emails that challenge the AI model. Correlating workflow performance with the key metrics provides insights into which automations deliver the most significant impact.
Making data-driven improvements is the core of optimization. Use the insights gained from metric tracking and workflow analysis to refine automation rules, retrain AI models with more specific examples, or adjust workflow logic. A/B testing different configurations (e.g., comparing two different AI prompts for summarization) can help determine the most effective approach based on measured outcomes. This iterative cycle of measurement, analysis, and refinement ensures continuous improvement in email management efficiency, contributing to the broader productivity gains envisioned by reports like McKinsey's analysis on AI's economic potential.
Common Challenges and Solutions
Implementing AI email automation, while powerful, can present certain challenges. Anticipating and addressing these proactively ensures smoother adoption and sustained performance.
Handling exceptions is a common requirement. No AI model is perfect, and workflows will inevitably encounter emails that don't fit predefined rules or classifications. The solution involves building fallback mechanisms, such as routing uncertain cases to a specific "Needs Review" folder or flagging them for manual inspection. Regularly analyzing these exceptions can provide valuable data for refining the AI model or adjusting workflow logic to handle similar cases automatically in the future.
Troubleshooting issues requires a systematic approach. Common problems include API connection failures, errors in workflow logic, or unexpected AI behavior. Utilize the logging and debugging tools provided by the automation platform to pinpoint the source of the error. Test individual steps of the workflow in isolation to identify the faulty component. Consulting platform documentation or community forums (like those for Zapier or Make.com) can often provide solutions to common technical problems.
Scaling automation workflows as volume or complexity increases requires careful planning. Ensure the chosen platform can handle the anticipated load and that workflows are designed modularly for easier maintenance and expansion. Avoid creating overly monolithic workflows; break down complex processes into smaller, interconnected automations. Regularly assess performance under load and optimize resource utilization to prevent bottlenecks as usage grows.
Future of AI Email Automation
The field of AI email automation is rapidly evolving, driven by advancements in machine learning and natural language processing. Observing emerging trends allows organizations to prepare for future capabilities and maintain a competitive edge in productivity.
Emerging trends suggest a move towards more proactive and predictive email management. Future AI systems may anticipate user needs, automatically scheduling meetings based on email context without explicit requests, or proactively archiving emails once related tasks are completed. Hyper-personalization in automated responses, tailored precisely to the recipient's context and history, is another area of development, potentially enhancing communication effectiveness as discussed in broader terms of how generative AI is changing work by HBR.
New tools and capabilities are constantly entering the market. Expect tighter integrations between email clients, AI assistants, and collaborative platforms, creating a more unified digital workspace. Advancements in multimodal AI could allow systems to understand content within images or attachments more effectively, enabling more sophisticated automation triggers and actions. The ability of AI to summarize complex threads or extract key decisions will likely become more refined.
Preparing for future developments involves fostering a culture of continuous learning and adaptation. Stay informed about new AI tools and platform updates through industry publications and vendor announcements. Encourage experimentation with new features in controlled environments to assess their potential value. Building flexible, modular automation architectures today will make it easier to integrate new capabilities as they become available, ensuring your email management strategy remains efficient and effective.
Conclusion
The challenge of email overload is a significant drain on productivity, but AI-powered automation offers a robust, data-driven solution. By leveraging intelligent tools for classification, prioritization, and even communication assistance, individuals and organizations can reclaim substantial amounts of time, improve responsiveness, and reduce the stress associated with inbox management. The measurable benefits, from reduced manual sorting time to faster response rates, underscore the value of integrating AI into email workflows.
To recap the key benefits, AI automation enables smarter email sorting and filtering, highlights priority communications effectively, assists with drafting replies and summarizing content, and integrates seamlessly with other productivity tools for streamlined processes. Implementing these systems transforms the inbox from a source of friction into an optimized component of your digital workflow. As we've discussed in sections like Measuring Success, the impact is quantifiable and significant.
Getting started involves a clear checklist:
- Assess your current email challenges and identify high-impact areas for automation.
- Select an appropriate AI automation tool or platform (Zapier, Make.com, etc.).
- Begin with basic workflows like Email Classification and Priority Management.
- Test rigorously and establish baseline metrics for performance tracking.
- Monitor performance, analyze data, and iteratively refine your workflows.
The next steps involve committing to this analytical approach. Start small, measure consistently, and gradually expand your use of AI email automation based on demonstrated results. By embracing these tools and techniques, you can achieve a more efficient, organized, and less stressful relationship with your inbox.
Additional Resources
For those looking to delve deeper into AI email automation, here are some valuable resources:
- Recommended Tools and Platforms:
- Zapier: Explore their AI tools and extensive integrations for building email workflows.
- Make.com (formerly Integromat): Discover advanced workflow automation capabilities for email and beyond.
- Further Reading:
- Harvard Business Review: How Generative AI Is Changing Work: Understand the broader context of AI's impact on productivity and work processes.
- McKinsey & Company: The Economic Potential of Generative AI: Gain insights into the significant economic and productivity implications of AI technologies.
- Community Support Options:
- Explore the community forums and help centers provided by platforms like Zapier and Make.com for peer support, troubleshooting tips, and workflow inspiration. Engaging with these communities can provide practical solutions and insights from other users implementing similar automations.